Cognitive and ontological modeling for decision support in the tasks of the urban transportation system development management [Electronic resource] / D. Parygin, N. Sadovnikova, A. Kravets, E. Gnedkova // IISA 2015 : Proceedings of the Sixth International IEEE Conference on Information, Intelligence, Systems and Applications, Corfu, Greece, 6–8 July 2015. – IEEE, 2015. – P. 1–5. – Mode of access : http://ieeexplore.ieee.org/xpl/articleDetails.jsp?arnumber=7388073 – DOI : 10.1109/IISA.2015.7388073
Danila Parygin
Natalia Sadovnikova
Alla Kravets
Elena Gnedkova
Abstract. Rapid changes in the urban environment as results of technological progress require new approaches to the development of transport infrastructure, which will increase the performance of the transport system, improve the quality of its operation and safety. It is necessary to maintain the quality of life, business and economical stability of the city. However, the development of the transport system or its radical transformation is a large-scale investment, which is not always easy to decide, especially in the face of uncertainty: lack of knowledge about the process and fluctuations in the economy. Deficient of transport services aspects study will inevitably lead to losses of life quality. Thereby the development of methods for optimizing the route network of public transport, which will link the demand and supply of transport services, is an urgent task. In this paper, we propose a new approach to the construction and refinement of the ontological model of knowledge representation about the city transport system using cognitive modeling.
Keywords. Public transportation; city; decision support; cognitive modeling; management of complex systems; ontology.
I. Introduction
The urban transportation system development is an important part of public policy, since it contains a number of solutions to social and environmental problems and significantly affects the quality of life in the city. First of all the urban building plan and urban development plan, patterns of forming demand for moving around the city, choose the best ways and routes of daily migration, optimize the amount of rolling stock of public transportation, timetables and so on should be taken into account to solve this task. Another problem lies in the fact that in Russia and in other countries the urban public transportation is traditionally unprofitable and cannot develop normally on its own economic base, to be «self sufficient» in financial terms. Transportation tariffs cover only 50-60% of operating costs despite the constant increase, while the remaining 40-50% comes from various external sources, mostly from local (regional) budgets [1].
However, in the world practice has accumulated rich experience in dealing with the inefficiency of passenger transportation and proposed various approaches to improve the functioning of urban transportation system.
There is the problem that the leaders of the municipalities do not have the tools for comprehensive analysis the experience and evaluation of its application under conditions of the city. The need to respond quickly to changing situations and taking into account a number of factors requires the use of technologies and methods based on modern approaches to processing and analysis of information and decision support.
A lot of researches are devoted to the issues of decision support in the development of urban transportation systems.
As a rule, they are based on the methods of simulation modeling or multi-criteria optimization. There are systems that are focused on the tasks of strategic planning of road infrastructure, evaluating the current and project state of traffic management («PTV Vision® VISSIM», Germany), modeling the demand for movement and predict traffic conditions (EMME4, Canada), the analysis of traffic flow (AIMSUN2, USA) and others.
All these systems are focused on specific tasks, while the ability to analyze the functioning of urban transportation system as a whole object for today has not been implemented.
The concept of intelligent decision support for managing the development of urban transportation system proposed in this paper is the result of an analysis of different approaches to the knowledge formalization and decision making under uncertainty organization [2]. Creation of an unified model of urban transportation system strategic planning development based on integrated models of knowledge representation and complex evaluation procedures that take into account the ambiguity and multi-criteria problems of choice managing impacts, provides new opportunities for solving the decision support problems.
II. The task of decision support for development management of urban transportation system
An urban transportation system is a complex system which united a number subsystems: transportation infrastructure, street and road network and communication, rolling stock, depots, parks and repair base, organizational and personnel subsystem, traffic management subsystem, legislation and others. The development management of urban transportation system in this paper is considered as a set of actions, selected on the basis of specific information and aimed at maintaining or improving the functioning of the system, increase competitiveness, accessibility and quality of passenger services, effective satisfaction of transportation demand in general.
Urban transportation system is used by various stakeholders who are pursuing conflicting interests. Among these groups can be distinguished population, the city administration and various business entities. It should be noted that interests of the population, government and business do not always lie in the same plane that frequently leads to additional problems [3].
Modern trends in the development of urban transportation systems are based on the concept of human-oriented management rather than transport. The concept of development urban transportation system chosen by the municipality determines the composition and value of indicators against which to assess the achievement of management objectives.
Decision support systems represent a specific class of automated information systems that support decision-making activities. These systems help professionals select or generate the correct alternative among many options when making responsible decisions, providing the necessary information. It uses different methods for the analysis workings and suggestions for decision support systems [4].
Terms of solving problems have been identified in accordance with the basic principles of the decision support systems development.
Variants of using the transportation system were determined by expert analysis. Decomposition of the goal carried out and thus implies:
− development of public transportation;
− development of road-transportation infrastructure;
− traffic management.
The task of making decisions on the development management of transportation system is formulated as follows: what actions are necessary to achieve the desired indicators of the system development taking into account the current situation and external environment factors? The most relevant methods of decision support should be used to effectively manage the transportation system.
Ontological approach is selected as the basic methodology in this study.
Currently, the decision support systems are widely using formal models expert knowledge represented by different levels of ontologies. Ontological approach to Decision Support Systems expands decision support in decision of the multitude problems classes in many different methods.
Decision support tasks that can be solved within the framework the ontological approach:
− support of a choice methods for solving problems;
− classification of information;
− integration of the diverse information;
− searching for relevant information for decision support;
− interpretation of the solution results.
Within the context of Decision Support Systems ontologies allow to fix and standardize the terminology of the subject area, arrange relevant for Decision Support information search, submit domain knowledge in formalized form suitable for machine processing. Using ontologies offers several advantages. Firstly, the use of a single ontological basis facilitates the integration of Decision Support Systems with external sources, including other Decision Support Systems. Secondly, ontologies can be directly involved in decision-making, i.e. to support case-based and rule-based systems. Thirdly, an ontology can act as a communication protocol between the different modules of systems, allowing system modules to be interchangeable and increasing flexibility.
In general, an ontology is the system of concepts of a subject area, which is represented as set of entities, connected by various relations. Ontologies are used for formal specification of concepts and relations that characterize a specific area of knowledge.
Identification of a subject area important factors (concepts) and links (relations), affecting the solution of the problem, is one of the most difficult tasks in the ontology construction.
Different approaches are proposed for solving this problem:
− textological methods (PEST and SWOT analysis);
− statistical methods (factor analysis, cluster analysis);
− approaches based on technology UML to OWL;
− cognitive modeling.
At present a knowledge representation is focused on semantic analysis and machine processing, research the context, which requires a certain degree of formalization. In such cases, the development and use of ontologies is common. Software used here (e.g., Protégé), and the process of designing and creating the ontology, however, are difficult for a non-experts. This leads to difficulties in situations collaboration of specialists in the development of ontologies. As a convenient system of human knowledge representation it is reasonable to use conceptual maps and, furthermore, cognitive modeling. Cognitive modeling is a tool that especially defined for application in the learning process: it is easy to be created, flexible and intuitive for people [5, 6, 7].
The approach to the choice of the ontology structure based on cognitive modeling is considered in this paper. The possibility of determining type of connection between concepts based on the cognitive model analysis is studied.
III. Building a cognitive model of development urban transportation system
Uncertainty of factors and their relationships is the feature of the cognitive modeling application to complex systems behavior identification. Values of the factors are most often represented as linguistic variables and interval estimates. Model structure can be changed during the study.
The modeled situation is described by fuzzy cognitive maps, which are weighted oriented graphs of cause-effect relationship that illustrate multiple links between the system factors, such as human behavior, administrative and technical changes, financial and economic alternatives. These maps are useful for identifying and representing the second-order effects. Further, the method of pulse processes, which falls under the category of dynamic methods, is used to obtain a forecast of development. Pulse process allows determining concept state in discrete time. Analyzing the state allows experts to receive a forecast of system state changes at realization of various management strategies and ambient changes [8].
The sequence of tasks for the implementation of this approach can be defined as follows:
1) identification of basic factors characterizing urban transportation system development;
2) identification of mutual influence of factors;
3) cognitive model construction;
4) formation of possible development scenarios;
5) defining criteria for evaluation scenarios;
6) scenario assessment and identification of the best scenario in terms of the criteria chosen;
7) analysis of development strategy based on chosen scenario [9, 10].
Basic factors selected to characterize the development of urban transportation system are shown in Table I.
TABLE I. Description the factors and relations of the cognitive model urban transportation system.
Designation | Name of factor | Direct impact on a factor | Inverse impact on a factor |
x1 | Informing traffic participants in transportation situation | x3, x31 | x17, x37 |
x2 | Comfort of travel in urban public transportation | x8, x36 | – |
x3 | Transportation accessibility using urban public transportation | x8, x9 | x34 |
x4 | Safety of urban public transportation | x8 | – |
x5 | Number of users of municipal urban public transportation in the total passenger traffic | x8 | – |
x6 | Number of users of private urban public transportation in the total passenger traffic | x8, x19 | – |
x7 | Density of routes urban public transportation to off-street road network | x3, x4, x10 | x17 |
x8 | Number of users of urban public transportation in the total passenger traffic | x13, x14, x17, x31 | x2, x40 |
x9 | Efficiency of the urban transportation system to meet the demand for transportation | – | – |
x10 | Coverage ratio of street and road network with routes urban public transportation | x19, x36 | x15 |
x11 | Monthly travel expenses as a percentage of the subsistence minimum | – | x9 |
x12 | Technical condition of the rolling stock urban public transportation | x2, x4, x36 | – |
x13 | Occupancy of the rolling stock urban public transportation | x14 | x2 |
x14 | Profit transportation company on a per-passenger of urban public transportation | x9, x41 | – |
x15 | Average distance to stopping point | x18 | x3 |
x16 | Average number of change on the route | x18 | x3 |
x17 | Average timeout of transportation | x13 | x3, x36 |
x18 | Costs passenger per kilometer using urban public transportation | x11, x34 | x21 |
x19 | Coefficient of the use minibuses on routes urban public transportation | x37 | x2, x4, x16, x17, x31 |
x20 | Density of the street and road network | x29, x31, x33 | – |
x21 | Number of passengers without travel benefits | x8, x34 | – |
x22 | Number of passengers with travel benefits | x5, x27 | x14, x21 |
x23 | Zoning territory of the city | x24, x26 | – |
x24 | Development the traffic management through the special equipment of street and road network | x1, x3, x29, x31, x39 | x37, x40 |
x25 | Development the system of paid parking on urban road network and transport hubs with intercept parking | x8, x29, x32 | x34 |
x26 | Development the system of additional fees from personal vehicles users in the city | x8, x32 | x34 |
x27 | Subsidization of municipal urban public transportation | x22, x41 | x18 |
x28 | Connectedness of the street and road network | x3, x29, x31 | x37 |
x29 | Level of capacity of the urban road network | x31 | x37 |
x30 | Quality of urban road-transport infrastructure | x3, x4, x29, x31 | x32, x36, x39 |
x31 | Transportation accessibility using personal vehicles | x9, x34 | – |
x32 | Costs passenger per kilometer using personal vehicles | x8, x11 | x21, x34 |
x33 | Budget expenditures for the maintenance and development of the street and road network | x24, x28, x30 | – |
x34 | Gain of number personal vehicles | x37, x39, x40 | – |
x35 | Fares on urban public transportation | x14, x18 | x8, x27 |
x36 | Costs urban companies of public transportation per kilometer | x35 | x9, x14 |
x37 | Formation of peak load the street and road network | x8, x17, x32, x39, x40 | x3, x31 |
x38 | Cost of fuel and power resources | x32, x36 | x34 |
x39 | Number of accidents | x37 | x4 |
x40 | Ecological load of urban environment | – | – |
x41 | Investments into development of urban public transportation | x2, x7, x12 | – |
Fragment of the graph of the cognitive model urban transportation system is shown in Fig. 1, and the fragment of the matrix mutual influences corresponding to the figure in Table II.
The urban transportation system model is focused on evaluating the effectiveness meet the demand for transportation by studying the balance of use public and personal transportation. In this case traffic management and task of management transportation infrastructure are the “external” (with the functions of service and regulation) in relation to the entire traffic flow.
Indication function of factors “Efficiency of the urban transportation system to meet the demand for transportation” and “Ecological load of urban environment” allows to carry out a comprehensive evaluation of the simulated situation. However, the inclusion in the model components of a lower level, thereby providing a tool for the selection and specification of their impact on the overall situation, is particularly important. At the same time the impact of changes in the transportation system, such as a “technical” factors that characterize the work public transportation are identified: key time intervals forming a common transportation accessibility or subjective factors emotional evaluation of comfort and safety.
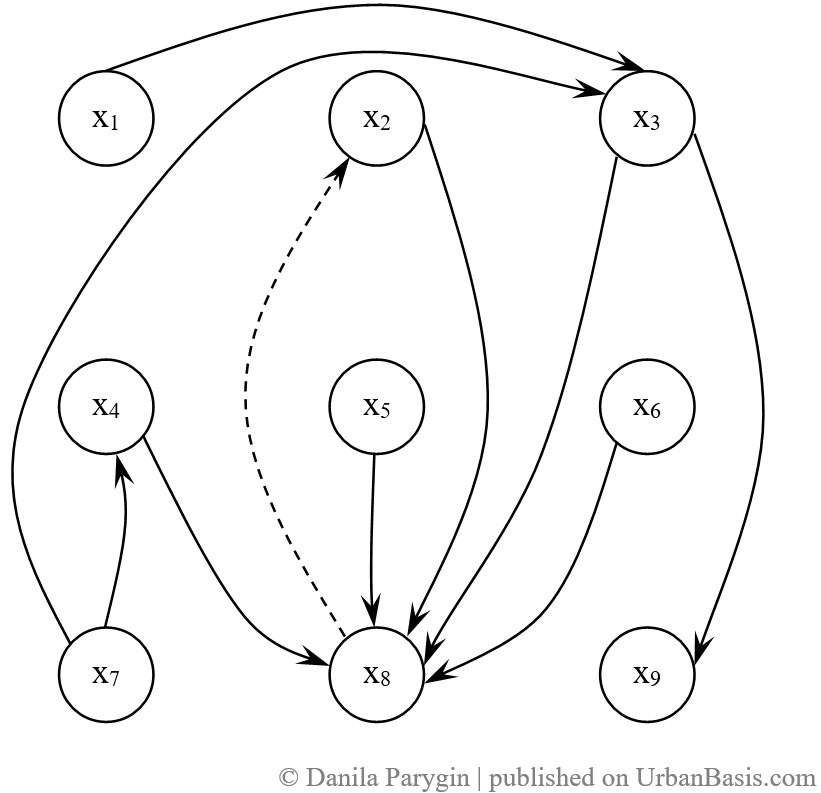
TABLE II. Fragment of the matrix mutual influences of the cognitive model urban transportation system/
x1 | x2 | x3 | x4 | x5 | x6 | x7 | x8 | x9 | |
x1 | 0 | 0 | +0.4 | 0 | 0 | 0 | 0 | 0 | 0 |
x2 | 0 | 0 | 0 | 0 | 0 | 0 | 0 | +0.2 | 0 |
x3 | 0 | 0 | 0 | 0 | 0 | 0 | 0 | +0.6 | +0.7 |
x4 | 0 | 0 | 0 | 0 | 0 | 0 | 0 | +0.1 | 0 |
x5 | 0 | 0 | 0 | 0 | 0 | 0 | 0 | +0.5 | 0 |
x6 | 0 | 0 | 0 | 0 | 0 | 0 | 0 | +0.5 | 0 |
x7 | 0 | 0 | +0.6 | +0.8 | 0 | 0 | 0 | 0 | 0 |
x8 | 0 | –0.5 | 0 | 0 | 0 | 0 | 0 | 0 | 0 |
x9 | 0 | 0 | 0 | 0 | 0 | 0 | 0 | 0 | 0 |
IV. Example of the ontological model development
First of all we have selected a set of attributes describing an urban transportation system with the appropriate perspective based on the analysis of cognitive maps. The attributes were defined for their possible values and evaluation of these values in accordance with the objectives was fulfilled.
For example, the development of urban public transportation should provide a safe and secure movement of the city residents with the lowest cost of financial and natural resources and with the least damage to the environment. The attributes descriptions fragment for the task “Development of public transportation” is given in Table III.
Besides for the different attribute values we can define alternatives – the decisions and actions to be taken to improve the situation. Fig. 2 shows an example of possible alternatives for the attribute “Average timeout of transportation”.
The result of this phase is the construction of the domain knowledge field, which describes the basic concepts and relationships between them, identified from the system of expert knowledge. Further expertise was formalized in the form of an ontology language OWL-DL.
Fragment of a hierarchy of ontological concepts:
1. Case – a precedent that describes the specific case of the implementation of any action. Contains a description and evaluation of the initial and final states of a municipal facility, as well as a set of actions that have been taken.
2. State is used to describe the state of the object as a set of parameter-value pairs.
2.1. Public transportation.
2.1.1. Headway.
2.1.1.1. Acceptable – sufficient frequency of traffic.
2.1.1.2. Unacceptable – too long period between the arrival of transport.
2.1.2. Transport safety.
2.1.3. …
3. Action – a list of possible actions to change the situation.
3.1. Maintenance of the public transportation.
3.1.1. Increasing the number of transport units on this route
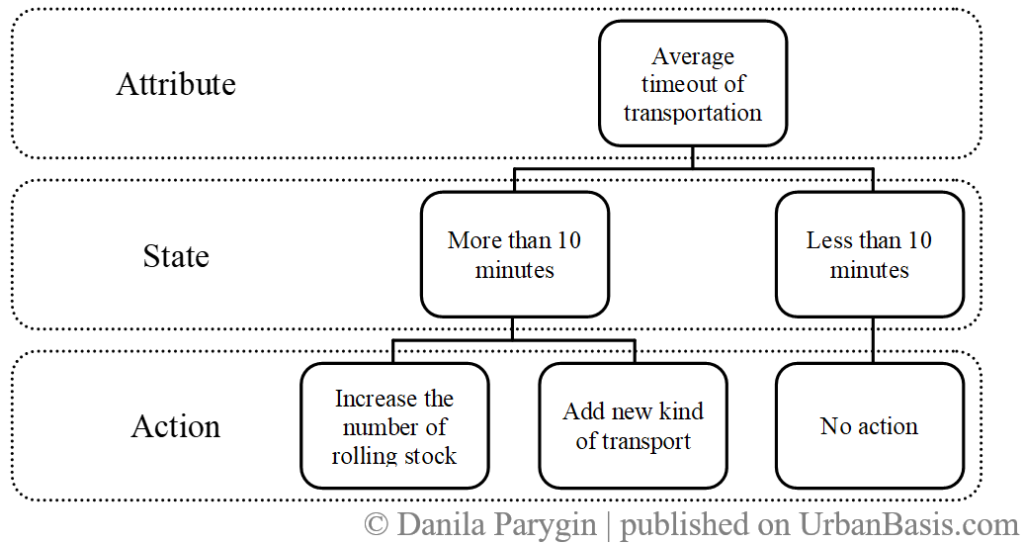
TABLE III. Description of the attributes and their values for the purpose of “Development of public transportation” [11].
Attribute | Acceptable | Unacceptable |
Density of the routes (the ratio of the total length of routes to the town square) | 2,0—3,5 km/km2 | Less than 2,0 km/km2 |
Average timeout of transportation | Less than 10 minutes | More than 10 minutes |
The technical condition of the rolling stock (RS) | More than half of the SS operated less than 10 years | More than half of the SS operated for over 10 years |
The coefficient of a straight path (route length divided by the distance between the start and end point of the route by air) | Less than 1,8 | More than 1,8 |
Transport accessibility | Half of the labor movement are carried out in less than 60 minutes | Half of the labor movement carried out during more than 60 minutes |
Number of passengers on the unit area of the vehicle | 3-5 people | Less than 3 or more than 5 |
Safety (number of accidents involving public transport) | Reducing | Increasing |
Transportation costs | Rout pay off | Route requires subsidies |
Interior temperature | From +10°С to +35°С | Below +10 °С, or above 35°С |
Monthly travel expenses as a percentage of the subsistence minimum | Less than 15% | More than 15% |
3.1.2. Organization overhaul of rolling stock.
3.1.3. …
3.2. …
4. Assessment – options to assess the situation from different perspectives.
4.1. Public transport.
4.1.1. Acceptable.
4.1.2 Satisfactory.
4.1.3. Unacceptable.
4.2. Road transportation infrastructure.
4.2.1. …
5. Mutual influence action – knowledge about the mutual influence on each other (mutual gain or loss).
V. Conclusion
The approach proposed in the paper helps developers to structure the basic ontological relations in the subject area and allows gradual expansion of ontology based on an analysis of cognitive model factors interactions. Integration, which is offered, lays the foundation for generalization to other areas and for the joint development of ontologies. Currently the developed models are used to structuring the information in problems of decision support in the development management of the urban transportation system. Patterns of urban transportation systems development have been studied in the framework of research. Studies have identified factors shaping ideas about the system, and indicators that characterize the occurring processes, structure and strength of system relations.
References
- I. Polyakova, “Transportation harmony”, http://www.transportrussia.ru/gorodskoy-passazhirskiy-transport/transportnaya-garmoniya.html
- B.Kh. Sanzhapov and N.P. Sadovnikova, “Agree objectives for environmental and economic feasibility of urban project subject to the restrictions on the values of the characteristics included in the system means in terms of fuzzy information,” Internet-vestnik VolgGASU, Construction and Architecture, vol. 21(40), pp. 151-159, 2011.
- N.P. Sadovnikova, D.S. Parygin, E.P. Gnedkova, B.Kh. Sanzhapov, and N.P. Gidkova, “Evaluating the sustainability of Volgograd,” The Sustainable City VIII, Proc. of the 8ht Int. Conf. on Urban Regen. and Sustain., Sec. 3, Putrajaya, Malaysia. WIT Press, 2013, pp. 279-290.
- D.S. Parygin, V.A. Kamaev, N.P. Sadovnikova, and A.Yu. Mironov, “The concept information-analytical system of management urban development,” Innovative Information Technology, Proc. Int. Sc.-Practic. Conf., Prague, Czech Republic, vol. 4. Moscow: MIEM HSE, 2013, pp. 205-213.
- A. Simon, L. Ceccaroni, and A. Rosete, “Generation of OWL Ontologies from Concept Maps in Shallow Domains,” Current Topics in Artificial Intelligence, Lecture Notes in Computer Science, vol. 4788, pp. 259-267, 2007.
- N.P. Sadovnikova, “Application of the cognitive modeling for analysis of the ecological and economical efficiency of the urban planning project,” Internet-vestnik VolgGASU, Civil Engineering Informatics, vol. 5(14), pp. 1-7, 2011.
- A. Korotkov, A.G. Kravets, Y.F. Voronin, and A.D. Kravets, “Simulation of the initial stages of software development,” Research Journal of Applied Sciences, vol. 9, No. 10, pp. 815-819, 2014.
- N.P. Sadovnikova, D.S. Parygin, E.P. Gnedkova, A.G. Kravets, A.V. Kizim, and S.S. Ukustov, “Scenario forecasting of sustainable urban development based on cognitive model,” ICT, Society and Human Beings 2013, Proc. of the IADIS Int. Conf. Prague: IADIS Press, 2013, pp. 115-119.
- D.S. Parygin, “Assesment the effectiveness management strategies of urban area based on scenario models,” Proc. of VSTU, Actual problems of management, computer science and informatics in technical systems, vol. 8(111), pp. 98-103, 2013.
- D.S. Parygin, N.P. Sadovnikova, and N.P. Gidkova, “Constructing trajectories territorial development based on methods of scenario forecasting” Internet-vestnik VolgGASU, Civil Engineering Informatics, vol. 8(24), pp. 1-9, 2012, http://vestnik.vgasu.ru/attachments/ParyginSadovnikova-2012_8(24).pdf
- N.P. Sadovnikova, E.P. Gnedkova, and D.A. Shirmanova, “The ontological knowledge representation model, supporting decision-making processes in the field of the city strategic planning,” Proc. of VSTU, Actual problems of management, computer science and informatics in technical systems, 2015, in press.