Models and methods for the urban transit system research / N. Sadovnikova, D. Parygin, M. Kalinkina, B. Sanzhapov, Trieu Ni Ni // CIT&DS 2015 : Proceedings of the First International Conference on Creativity in Intelligent Technologies & Data Science, Volgograd, Russia, 15–17 September 2015. – Springer IPS, 2015. – CCIS 535. – P. 488–499. – DOI : 10.1007/978-3-319-23766-4_39
Danila Parygin
Natalia Sadovnikova
Maria Kalinkina
Bulat Sanzhapov
Trieu Ni Ni
Abstract. This paper describes the approaches to analyzing the effectiveness of the urban transit system. Research in this area almost focuses on the intersection of sciences: urban development, economics, ecology, sociology, and others. It is, therefore, important to use models that allow to perform analysis from different perspectives. It is necessary to take into account a large number of diverse factors which effect on the performance of the transportation system, its operation quality, safety, etc. For the conceptual analysis, it was provided to consider ongoing processes on the cognitive model. Decision support fuzzy methods are used to match the goals and possibilities of the transport system.
Keywords: city, transportation system, public transportation, decision support, cognitive modeling, fuzzy methods
1 Introduction
The urban transit system (UTS) is at the forefront in ensuring the proper functioning of life support systems, the development of all urban subsystems, business and tourism. The level of transportation accessibility largely determines the quality of life. The complexity of urban transit systems depends on the traffic increase, high environmental standards and the strict technical regulations operation of the vehicles. Modernization of the urban transit system is carried out with a view to improving the efficiency and profitability, quality and accessibility of transportation for the citizen. In this connection, it is necessary to solve a number of relevant tasks: identifying needs for transportation services, the determination of the factors influencing their satisfaction, identifying dependencies between the factors, assessment of the effectiveness of measures to improve the quality of transportation services and others.
2 Urban Transit System Development Modeling
When generating evidence-based approach for management decision-making to modernize the urban transit system it is necessary to use methods to create and analyze various alternatives and evaluate their effectiveness. Usually, for this use computer simulation technology.
Simulation allows researchers compare the effect of the transportation system operation and the cost of this operation, as well as the needs of society and the degree of their satisfaction. Moreover, these technologies make it possible to solve many other problems related to planning activities to create an effective urban transit system.
Formally, the mathematical models used for the analysis and design of urban transit system can be divided into three groups:
- forecast;
- imitation;
- optimization.
Again each group of models can be associated with a specific range of tasks:
- transit planning;
- traffic organization;
- optimization of transportation process.
The problems related to the planning are particularly important. City management can be effective only if operation of urban systems analyzed, problems identified and development opportunities specified. There are determine the conditions for reasonable choice of place for the construction of new enterprises and residential areas.
“Under what conditions will be a balance between the demand for transportation and transport supply” is one of the main questions that most researchers try to address. There are many approaches to solving this problem. Most of them are based on the analysis of statistical information on the movement of residents [1,2,3].
In [4] is considered the process of regulation of urban passenger transportation in the conditions of conflict interest passengers, transportation operators, and municipal authorities.
The most effective transportation models are derived from the integration of GIS-technologies and data analysis. This approach was implemented in «PTV Vision® VISUM» (Germany) [5]. Model that implemented in the system allows to obtain a holistic representation and scenario forecasts of the behavior transportation system at the macro level (city, region), carry out the calculation of the real functioning of the transportation system in different conditions [6]. Transportation demand is determined on the basis of data on the distribution of inhabitants, jobs, and job training, as well as jobs in the service sector by the transportation areas of the city. It should be noted that obtaining these data can be provided by a specially organized system for collecting traffic data at the federal, regional and local levels. Such system a long time and successfully operates in Germany [7], but this practice is not in Russia.
In addition, obtaining a complete matrix of correspondences measured recovery method from the traffic load on the segments of the network requires a large amount of information for the entire network of the city [8].
Many tasks cause considerable difficulties despite the successful experience of the simulation application for UTS planning development. First of all, it is the task which align objectives and linking them with the overall objectives of territorial development. It should also take into account that transportation systems are working in conditions of substantial uncertainty that is formed as the result influence various factors and actors on the situation. When building the UTS model should be considered fuzziness of the available information, stakeholders contradictions, and multicriteria solutions of optimization problems. In this regard, we consider an approach based on a combination of cognitive and fuzzy models.
Cognitive modeling is used for working with semistructured (weakly formalized) systems, and can be used in areas where there has never made attempts perform the simulation. In such systems, you can’t use the traditional econometric approach to analyze processes and to develop complex (i.e., dealing with different aspects of the studied system) solutions. With the help of cognitive modeling, we can obtain new knowledge about the possible development of the urban transit system. This knowledge can significantly reduce the uncertainty in future conditions estimation and reduce the number of unreasonable decisions taken by the city authorities. The cognitive map analysis allows to consider the impact of various factors on the development of the situation and taking into account the views of all interested parties. This approach is very effective in the pre-planning stage when necessary to analyze the interaction between difficult formalizable and measurable factors and finally to make decision on the system structure.
The simulated situation is described by means of fuzzy cognitive map [9], which is graph of cause-and-effect relations and are illustrated the multiple links between different factors and, therefore, are particularly useful for identifying and mapping the impacts of second order (indirect, synergies, etc.).
Key factors are the subject of research at the graph nodes. The list of sets N={Cn, Lij, Sgij, Wij}, where {Cn} – the vertex set (concepts); {Lij} – the set of cause-and-effect relations between concepts; {Sgij} – the character set of associations (+, –); {Wij} – the system set of associations (strong, medium, weak, etc.) is used in fuzzy cognitive maps. Each concept Ci is described by one or more variable conditions that characterize the state of the concept (qualitatively or quantitatively).
Based on the cognitive model we can be built scenario models and analyze planned solutions [10,11,12,13,14]. In this paper, a cognitive model is applied for structuring subject domain and conceptual analysis. The object modeling – the urban transit system as a set of communications of all types of transportation with their facilities, devices, engineering equipment, including control systems, management and organization of the movement of vehicles and pedestrians [15].
The objectives of simulation:
- structuring knowledge of the urban transit system and present them in a formalized form;
- identification of consensus view on the situation in the studied area and definition of contradictions;
- analysis of the mutual influence of factors, which are characterizing the situation;
- preparation of information for structure-targeted analysis the situation.
First Stage. Identification of basic factors characterizing urban transportation system development. This stage is aimed at limiting the subject area within the objectives and the formation of a unified conceptual system for the participants of this process. The quality of the urban transit system functioning will be assessed in the position to achieve significant effects on meeting the demand for movement, reduce operating costs, improve safety and comfort and to reduce the environmental burden.
Therefore, the three factors that determine the sustainable development of transportation system have been identified: reliability, efficiency, and safety. The reliability of transportation system is characterized by the degree of conformity to norms transportation accessibility and regularity, a guaranteed level of service and safety of trips.
The efficiency transportation system is determined primarily the ratio of income and expenses of transportation enterprises. The main objective of urban transit system is to ensure the mobility of the population, so the efficiency of transportation system will always be determined by a certain balance between the conflicting demands of the economy and the needs of residents. Urban passenger transportation is traditionally unprofitable and some of the funds needed to provide transportation are covering by local budgets. In such circumstances, the indicators related to the efficiency of spending, should be considered.
Security is a basic criterion for determining the quality of the transportation system. In this case, consider two aspects of security – technological and ecological. The most significant factors influencing the development of urban transit system were selected on the basis the analysis of task and different sources [16,17,18]:
- Q – Quality of UTS;
- x1 – Traffic Reliability;
- x2 – Transportation System Efficiency;
- x3 – Transportation System Safety;
- x4 – Meet the Demand for Services;
- x5 – Comfortable Transportation;
- x6 – Share of Users Urban Public Transportation in the Total Passenger Traffic;
- x7 – Transportation Prime Costs;
- x8 – Technological Safety;
- x9 – Environmental Safety;
- x10 – Transportation Accessibility;
- x11 – Movement Time to Stopping Point;
- x12 – Informing Passengers;
- x13 – Service Quality;
- x14 – Regularity;
- x15 – Vehicles Fullness;
- x16 – Vehicles Condition;
- x17 – Share of Sustainable Transport;
- x18 – Share Budget Expenditure on Environmental Safety;
- x19 – Route Network Density;
- x20 – Average Number of Change on the Route;
- x21 – Quality of Management;
- x22 – Fares;
- x23 – Costs of Transportation per Citizen;
- x24 – Driver Qualification;
- x25 – Quality Road-Transport Infrastructure;
- x26 – Traffic Organization Level.
Second Stage. Identification the factors mutual influence.
The cognitive model factors mutual influence can be determine by the dependencies based on the quantitative or qualitative estimates (obtained from the experts in this case). The relationship between the factors can be defined using the correlation coefficient in the case where there are proper quality statistics. Otherwise, we should apply evaluations based on the expert’s polls. Mutual influence of factors selected to characterize urban transportation system development are shown in Fig. 1 and Fig. 2.
Third Stage. Cognitive model construction.
The above model of urban transportation system is focused on evaluating the effectiveness meet traffic demand by examining the use of the public and private road transport balance. It is particularly important to have an inclusion possibility in the model the lower level factors. Thus, tools for selection and specification of their impact on the overall situation are provided. At the same time we will identify the impact transportation system transformation on, for example, the “technical” factors related to the passenger transportation operation: the key time slots that form the common transportation accessibility or the subjective factors the comfort and safety emotional evaluation.
3 Fuzzy Model for Matching Transportation System Development Objectives
As a rule, cognitive analysis tool is used to evaluate the influence of individual factors on the possibility of achieving the selected goals. It is difficult to conduct a combination of factors influence study and to determine the optimal of the studied systems values characteristics using only cognitive analysis.
Information obtained as a result of cognitive analysis can be used to determine the structure of the graph optimum requirements to the quality indicators and characteristics of the studied system. The intersection of factors and the visualization of the cognitive model factors in the values of the hierarchical model criteria are necessary to provide for the integration of these models.
Transportation system development concept identification has much uncertainty in the requirements and capabilities the objectives realization. Therefore, the task of determining the optimal requirements for quality indicators of the system based on the use of the principle of maximizing solution to the problem with fuzzy objectives and fuzzy restrictions [19]. This problem is non-linear with a complex structure restrictions. The standard method of nonlinear programming for its decision is not to allow in some cases the absence of an analytical description of communication functions between the indices of adjacent layers of the graph, as well as large dimension of the set vertices.
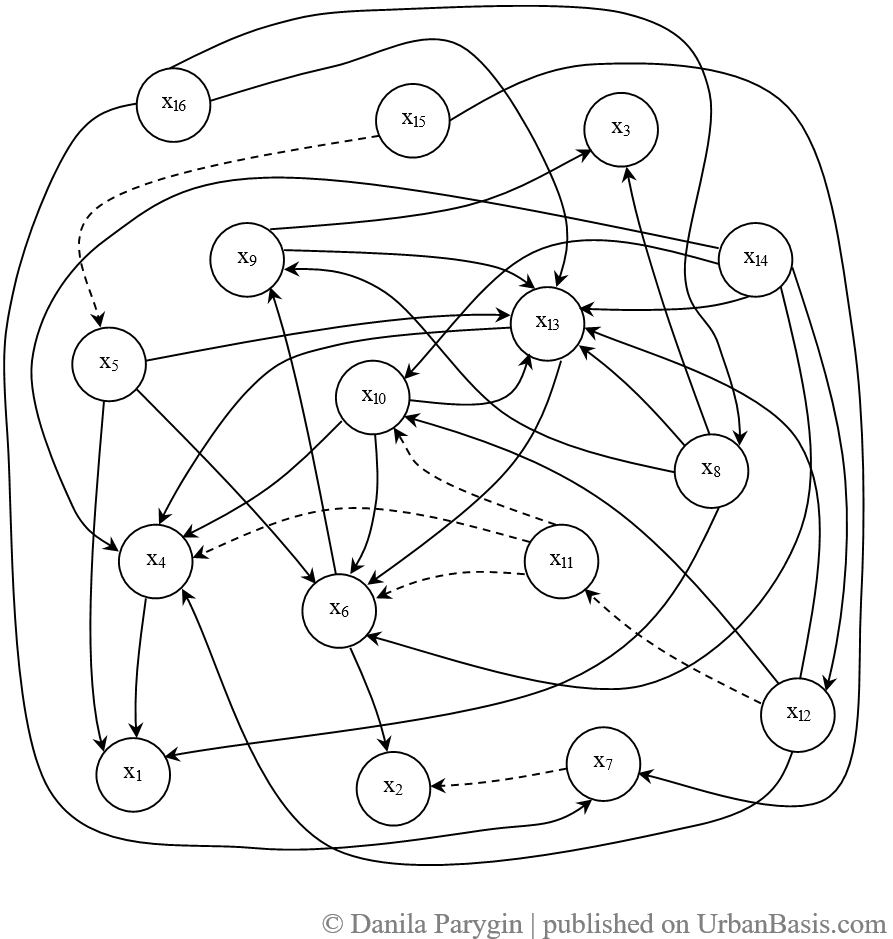
x1 | x2 | x3 | x4 | x5 | x6 | x7 | x8 | x9 | x10 | x11 | x12 | x13 | x14 | … | x26 | |
x1 | 0 | 0 | 0 | 0 | 0 | 0 | 0 | 0 | 0 | 0 | 0 | 0 | 0 | 0 | … | 0 |
x2 | 0 | 0 | 0 | 0 | 0 | 0 | 0 | 0 | 0 | 0 | 0 | 0 | 0 | 0 | … | 0 |
x3 | 0 | 0 | 0 | 0 | 0 | 0 | 0 | 0 | 0 | 0 | 0 | 0 | 0 | 0 | … | 0 |
x4 | 0,7 | 0 | 0 | 0 | 0 | 0 | 0 | 0 | 0 | 0 | 0 | 0 | 0 | 0 | … | 0 |
x5 | 0,4 | 0 | 0 | 0 | 0 | 0,5 | 0 | 0 | 0 | 0 | 0 | 0 | 0,8 | 0 | … | 0 |
x6 | 0 | 0,8 | 0 | 0 | 0 | 0 | 0 | 0 | 0,6 | 0 | 0 | 0 | 0 | 0 | … | 0 |
x7 | 0 | – 0,5 | 0 | 0 | 0 | 0 | 0 | 0 | 0 | 0 | 0 | 0 | 0 | 0 | … | 0 |
x8 | 0,7 | 0 | 0,7 | 0 | 0 | 0 | 0 | 0 | 0,3 | 0 | 0 | 0 | 0,5 | 0 | … | 0,2 |
x9 | 0 | 0 | 0,3 | 0 | 0 | 0 | 0 | 0 | 0 | 0 | 0 | 0 | 0,2 | 0 | … | 0,1 |
x10 | 0 | 0 | 0 | 0,7 | 0 | 0,7 | 0 | 0 | 0 | 0 | 0 | 0 | 0,4 | 0 | … | 0 |
x11 | 0 | 0 | 0 | – 0,2 | 0 | – 0,5 | 0 | 0 | 0 | – 0,4 | 0 | 0 | 0 | 0 | … | 0 |
x12 | 0 | 0 | 0 | 0,2 | 0 | 0 | 0 | 0 | 0 | 0,4 | – 0,2 | 0 | 0,6 | 0 | … | 0,1 |
x13 | 0 | 0 | 0 | 0,4 | 0 | 0,7 | 0 | 0 | 0 | 0 | 0 | 0 | 0 | 0 | … | 0,2 |
x14 | 0 | 0 | 0 | 0,3 | 0 | 0,3 | 0 | 0 | 0 | 0,5 | 0 | 0,6 | 0,6 | 0 | … | 0 |
x15 | 0 | 0 | 0 | 0 | – 0,7 | 0 | 0,1 | 0 | 0 | 0 | 0 | 0 | 0 | 0 | … | 0 |
x16 | 0 | 0 | 0 | 0 | 0 | 0 | 0,7 | 0,7 | 0 | 0 | 0 | 0 | 0,5 | 0 | … | 0 |
x17 | 0 | 0 | 0 | 0 | 0 | 0 | 0 | 0 | 0,9 | 0 | 0 | 0 | 0,1 | 0 | … | 0 |
x18 | 0 | 0 | 0 | 0 | 0 | 0 | 0 | 0 | 0,4 | 0 | 0 | 0 | 0 | 0 | … | 0 |
x19 | 0 | 0 | 0 | 0 | 0 | 0 | 0 | 0 | 0 | 0,7 | – 0,9 | 0 | 0 | 0,3 | … | 0 |
x20 | 0 | 0 | 0 | 0 | – 0,6 | 0 | 0 | 0 | 0 | 0 | 0 | 0 | 0 | 0 | … | 0 |
x21 | 0 | 0 | 0 | 0 | 0 | 0 | 0 | 0 | 0 | 0 | – 0,1 | 0,2 | 0,6 | 0,6 | … | 0,2 |
x22 | 0 | 0 | 0 | 0 | 0 | 0,5 | 0 | 0 | 0 | 0 | 0 | 0 | 0 | 0 | … | 0 |
x23 | 0 | 0 | 0 | 0 | 0 | 0 | 0 | 0 | 0 | 0,5 | 0 | 0,3 | 0,4 | 0 | … | 0 |
x24 | 0 | 0 | 0 | 0 | 0,3 | 0 | 0 | 0,7 | 0 | 0 | 0 | 0 | 0,5 | 0 | … | 0,1 |
x25 | 0 | 0 | 0 | 0 | 0 | 0 | 0 | 0,7 | 0 | 0 | 0 | 0 | 0 | 0 | … | 0,7 |
x26 | 0,4 | 0 | 0,4 | 0 | 0 | 0 | 0 | 0,8 | 0 | 0,6 | 0 | 0 | 0 | 0 | … | 0 |
In this regard, the use of an approach based on the decomposition of the original problem is of interest [20,21]. The considered problem of large dimension non-linear programming can be reduced to the task sequence of smaller dimension under certain assumptions about the structure of the relationship between the characteristics and kind of used membership functions.
Assumptions about the monotony of communication functions in the system and quasi-concavity membership functions of fuzzy variables are introduced.
The graph is a generalization of the objectives and targets tree for the case where the connection between vertices are described by nonlinear functions. Graph G=(X,E,F) is introducing to describe the interactions in the system, where
X – set of vertices;
Е – set of arcs

F – relationship set.
The xi value of Xi characteristic is dependent on the xj value of Xj characteristic like
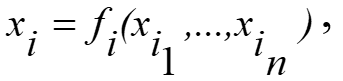
the fi functions are monotonically (non strictly) increasing in each of its arguments.
Requirements for the quality indicators defined in the form of fuzzy sets

Let us assume that values q1,…,q3, of quality indicators
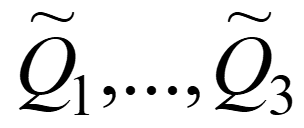
depend only on the values of the top-level factors.
It is expected that the membership functions of the parameters “Reliability”, “Efficiency” and “Safety” are monotonically (non strictly) increasing in each of its arguments and have a similar appearance (Fig. 3).
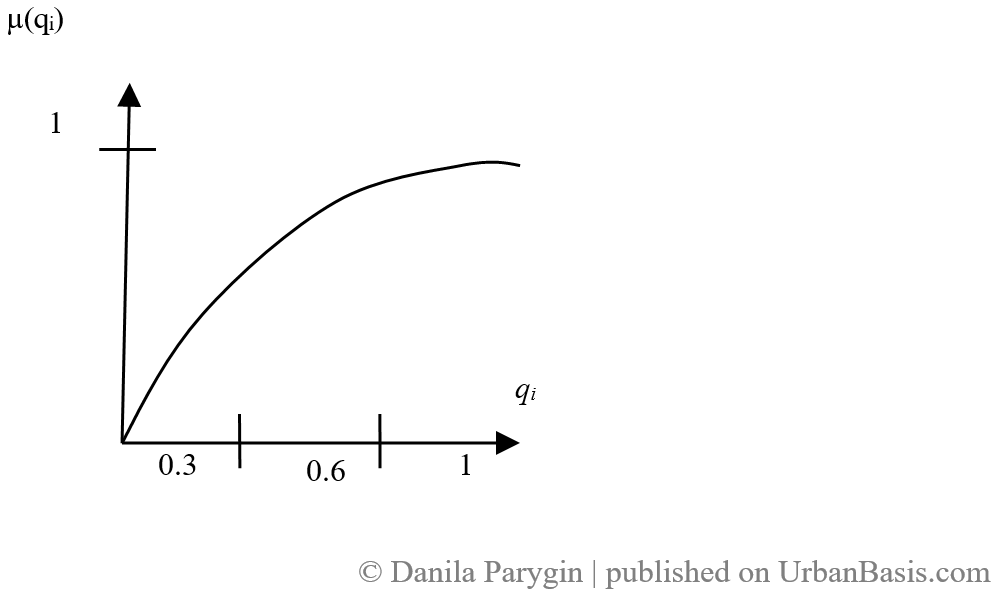
Possibilities the lower levels characteristics implementation are also modeled in the form of fuzzy sets

There
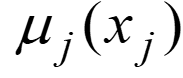
– the possibility that the xj variable can take appropriate value.
Examples of corresponding membership functions for some of the factors are listed below (Fig. 4). So the highest possibility that the fares will be in the range of from 20 to 50 rubles. Membership functions based on an analysis of information on the city of Volgograd.
The model presented in Fig. 5, was constructed to evaluate the urban transit system quality.
In order to determine communication functions we will use the information gathered at the stage of cognitive analysis. Weight factors in communication functions are determined in accordance with the matrix mutual influences (Fig. 2).
For example, the dependence of the motion regularity on the route network density and management can be expressed by the formula:

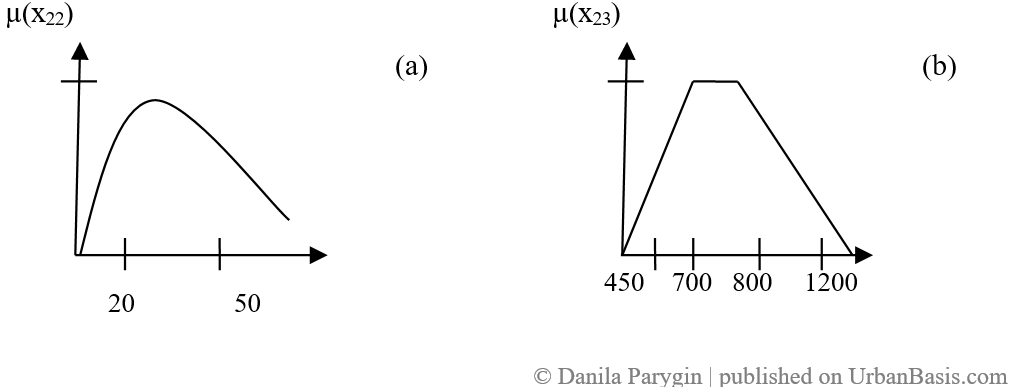
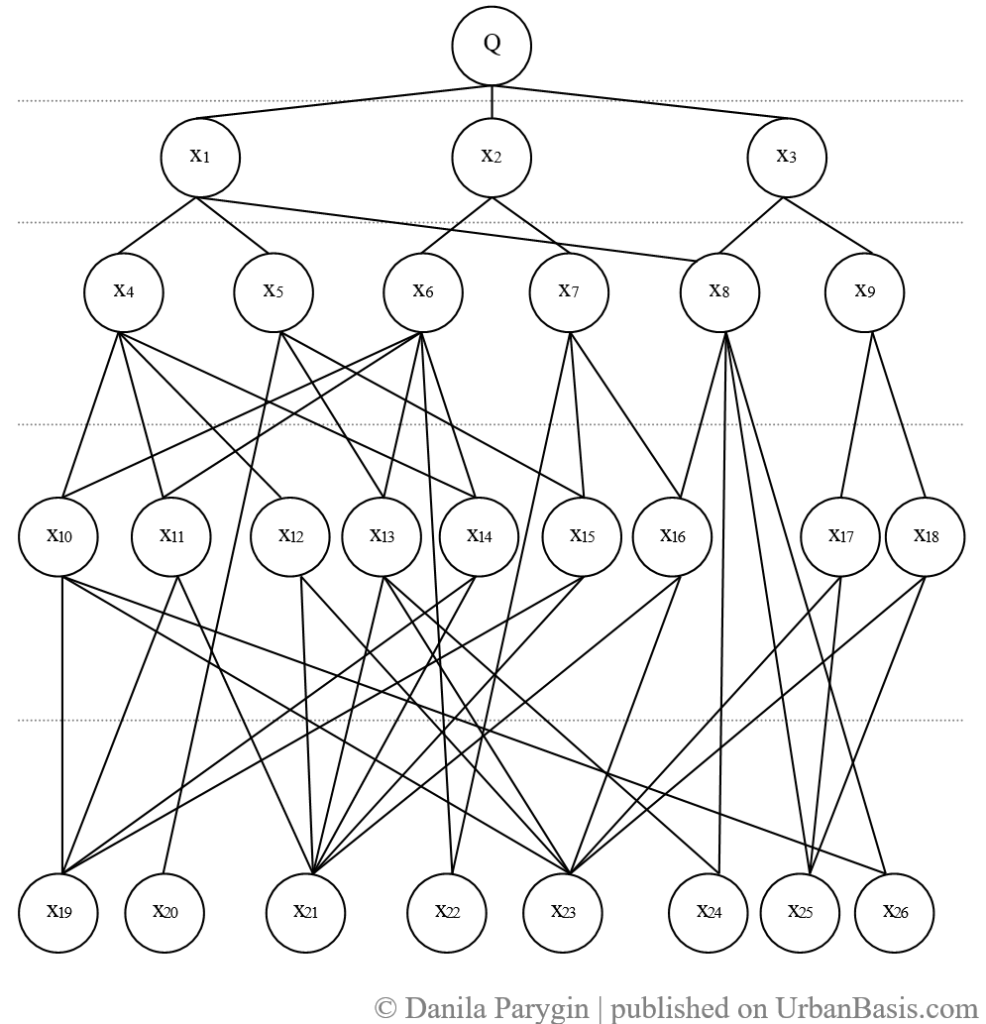
Method element wise division [20] involves computation capabilities of starting with the factors of penultimate level in accordance with the principle of generalizing LA Zadeh [19], based on the possibility for all factors Xj.
Ability to achieve the required characteristics for all values Xj, determined from:

where

The ability to achieve objective


As a result of solving the problem are the optimal requirements for system characteristics, quality indicators and assessment of achieving the goal:

The results of solving the problem are shown in Fig. 6.
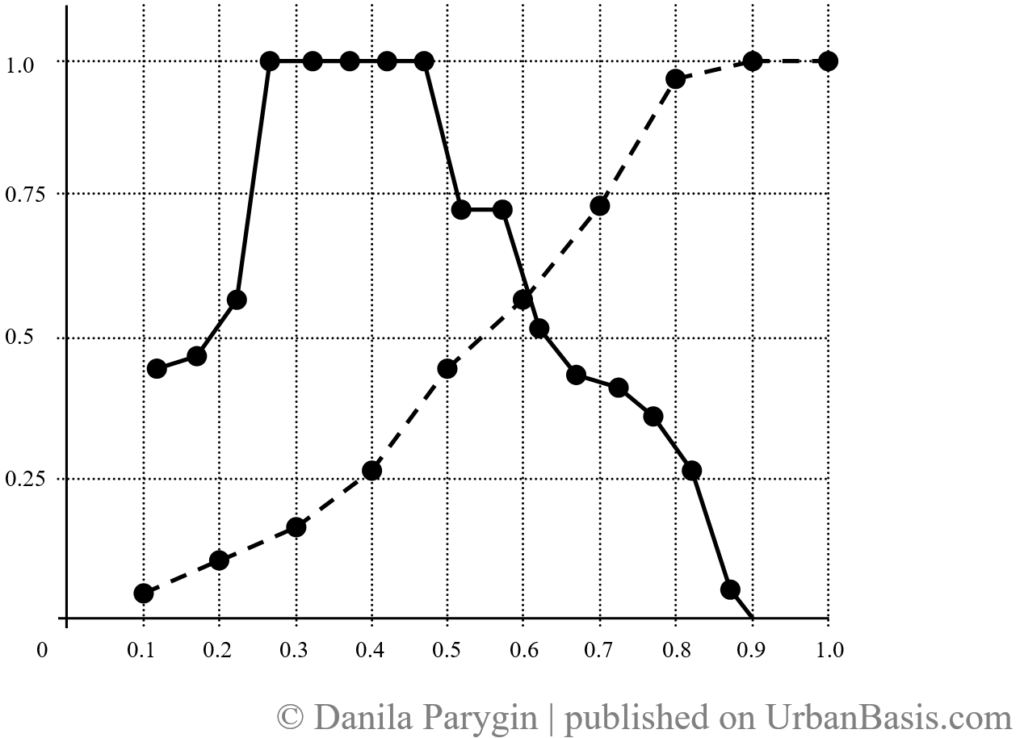
The intersection point of the membership functions graphs determines the degree of goal achievement. Therefore, 0.55 is the maximum possible value of the integral indicator of urban transportation system quality with the limits set on the possibility of the lower levels indicators implementation. In addition, the corresponding values of this lower level index factors could also be set.
4 Conclusions
Cognitive analysis and fuzzy modeling technologies integration can solve the problem of align objectives in dealing with the choice of strategies for the urban transit system development, and to obtain quantitative characteristics for the evaluation of alternative solutions.
Besides that the method presented in the paper can be used for:
- evaluation of the urban transit system performance;
- planning measures to improve the urban transit system;
- comparison of different variants of modernization projects the urban transit system;
- creating a basis for design making.
Acknowledgements. This research was supported by RFBR (projects № 13-07-00219-a, 15-07-03541-a) and Ministry of Education and Science of the Russian Federation within the frameworks of the project part of the Government statement (project № 2.1917.2014/K).
References
- Martin, W.A., McGuskin, N.A.: Travel Estimation Techniques for Urban Planning. National Cooperative Research Program Report 365. Transportation Research Board. National Research Council, Washington, DC (1998)
- Yakimov, M.R.: Mathematical modeling of the distribution of transportation demand in the urban transit system. Transport: science, technology, management (in Russian). 10, 7–13 (2010)
- Loze, D.: Modelling of transportation supply and demand for passenger and service transportation — an overview of modeling theory. Organization and safety of traffic in large cities. Proceedings of the Seventh International scientific-practical conference (in Russian), pp. 544. St. Petersburg (2006)
- Koryagin, M.E.: The equilibrium model of urban passenger transportation in the context of the conflict of interest (in Russian). Nauka, Novosibirsk (2011)
- PTV Vision Traffic Software, http://www.traffic-inside.com/ptv-vision/
- Ushchev, F.A.: Methodical and practical aspects of the application of transportation models in the planning of transportation infrastructure cities and regions of Russia. Conference “Improving the system of collection and analysis of information on the conditions of the accidents” (in Russian), pp. 111–120. St. Petersburg (2009)
- Astapenko, A.V., Apel’t, F., Semenov, S.A.: Methods of collecting traffic information and decision-making to create new roads and public transportation system – the German experience. Socio-economic problems of development urban transportation systems and their areas of influence. Proceedings of the X International (thirteenth Ekaterinburg) Scientific conference (in Russian), pp. 60–67. Izdatel’stvo AMB, Ekaterinburg (2004)
- Postnov, S.N., Kuznetsov, S.N., Loginov, P.V., Shirobakin, S.Ye., Byshov, N.V., Usenskiy, I.A., Yukhin, I.A., Yarusova, A.A.: Technology of creation the informational transportation model of the city, including existing and planned transportation networks (in Russian), http://www.uecs.ru/logistika/item/1591-2012-10-12-05-39-29
- Kosko, V.: Fuzzy Cognitive Maps. International Journal of Man-Machine Studies. 24, pp. 65–75 (1986)
- Chen, S.M.: Cognitive-map-based decision analysis based on NPN logics. Fuzzy Sets and Systems. 71, pp. 155–163 (1995)
- Kulba, V.V., Kononov, D.A., Kosyachenko, S.A., Shubin, A.N.: Methods of formation scenarios of socio-economic systems (in Russian). SINTEG, Moscow (2004)
- Parygin, D.S., Sadovnikova, N.P., Gidkova, N.P.: Constructing trajectories territorial development based on methods of scenario forecasting. Internet-vestnik VolgGASU, Civil Engineering Informatics (in Russian), vol. 8(24), pp. 1–9, http://vestnik.vgasu.ru/attachments/ParyginSadovnikova-2012_8(24).pdf
- Sadovnikova, N.P., Parygin, D.S., Gnedkova, E.P., Kravets, A.G., Kizim, A.V., Ukustov, S.S.: Scenario forecasting of sustainable urban development based on cognitive model. ICT, Society and Human Beings 2013. Proc. of the IADIS Int. Conf., pp. 115–119. IADIS Press (2013)
- Sadovnikova, N.P., Parygin, D.S., Gnedkova, E.P., Sanzhapov, B.Kh., Gidkova, N.P.: Evaluating the sustainability of Volgograd. The Sustainable City VIII. Proc. of the 8ht Int. Conf. on Urban Regen. and Sustain., pp. 279–290. WIT Press (2013)
- Recommendations for the modernization of the urban transit system MDS 30-2.2008 (in Russian), http://tehnorma.ru/normativbase/54/54029/ index.htm
- Gradoteka (in Russian), http://gradoteka.ru/city/volgograd/detail/ byudzhet/byudzhet-na-gorozhanina-c/rashody-na-transport-na-gorozhanina
- Papacostas, C.S., Prevedouros, P.D.: Transportation Engineering and Planning. Prentice Hall (2001)
- Sadovnikova, N.P., Gnedkova, E.P., Shirmanova, D.A.: The ontological knowledge representation model, supporting decision-making processes in the field of the city strategic planning (in Russian). Proc. of VSTU, Actual problems of management, computer science and informatics in technical systems (2015)
- Bellman, R., Zadeh, L.A.: Decision-making in vague terms. Questions of analysis and decision-making procedures (in Russian), pp. 172–215. Mir, Moscow (1976)
- Makeyev, S.P., Pitsyk, V.V., Poludenko, V.A.: Harmonization of the development goals of large technical systems capable of implementing their characteristics with fuzzy initial information. Proceedings of the Academy of Sciences of the USSR, Technical Cybernetics (in Russian). 5, pp. 124–132 (1991)
- Sanzhapov, B.Kh., Sadovnikova, N.P.: Agree objectives for environmental and economic feasibility of urban project subject to the restrictions on the values of the characteristics included in the system means in terms of fuzzy information. Internet-vestnik VolgGASU, Construction and Architecture (in Russian). 21(40), pp. 151-159 (2011)